Research Interests
My research sits at the intersection of AI, digital health, and human-computer interaction. I develop AI systems that use data from everyday technologies such as smartphone and wearables to understand and improve mental health in real-world settings. My work is highly interdisciplinary, and I collaborate with psychologists, clinicians, computer scientists, business professors, neuroscientists, and other researchers to tackle complex challenges in mental health and wellbeing.
Current Research Areas:
➊ AI-Driven Mental Health Detection: Developing novel approaches like depression detection through smartphone images and behavioral sensing
➋ Large-Scale Longitudinal Studies: Leading multi-year studies, including the longest mobile sensing study to date (5+ years tracking college students)
➌ AI-Based Adaptive Intervention Systems: Creating intelligent systems that learn from user behavior and adapt interventions in real-time, including LLM-powered platforms that deliver personalized mental health support based on behavioral context and user needs
My work addresses the growing mental health crisis by making support more accessible, objective, and timely. By leveraging everyday technology and adaptive AI, we can detect early warning signs and provide personalized interventions that evolve with each individual’s changing needs and circumstances. This research has the potential to improve mental health outcomes for millions and advance our fundamental understanding of human behavior, AI systems, and the complex relationship between technology and wellbeing.
News & Updates
→ 🎉 June 2025: Happy to share that I’ll be joining UVA as an Assistant Professor of Computer Science starting Fall 2025!
→ May 2025: Two recent papers acceptance/publications: survey paper on passive sensing in the workplace published at HCII and paper on AI-based productivity agent accepted at CSCW 2025.
Earlier:
→ 🏆 Received Stanford HAI Postdoctoral Fellowship
→ MoodCapture study featured on iHeartRadio and major media outlets for breakthrough in smartphone-based depression detection
→ Released the College Experience Dataset – the largest public mobile sensing dataset for mental health research (5 years, 200+ students)
About me
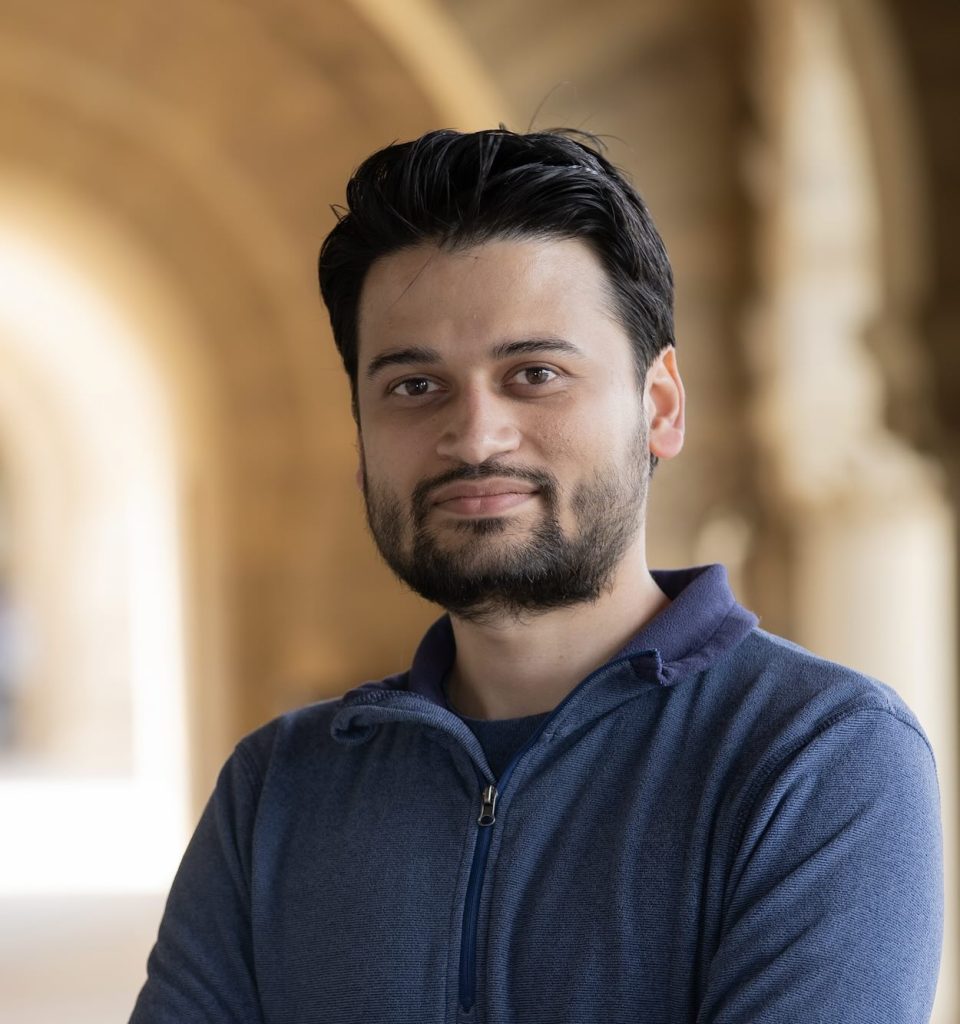
I’m an incoming Assistant Professor in the Computer Science Department at UVA.
Background:
▶ HAI Postdoctoral Fellow, Stanford University (2024-2025)
▶ PhD in Computer Science, Dartmouth College (2024)
📧 Email: sknepal@virginia.edu
🏢 Office: Rice Hall 104
85 Engineer’s Way
Charlottesville, VA